Reporte Interactivo: https://bit.ly/2SxBY4H
Por Hector Villafuerte, Julio 2021, https://twitter.com/hectorv_com
Resumen:
En las regiones excluyendo Lima, la distribución de votos para Fuerza Popular no es consistente en la población. Como lo muestra la siguiente figura, la distribución de votos para Fuerza Popular tiene una forma normal, cuando ambos personeros están presente durante el escrutinio. Pero cuando únicamente el personero de Perú Libre está presente en el escrutinio, la curva es extremadamente sesgada. Lo que no es consistente para la misma población. Esto muestra resultados diferentes en la votación cuando se segrega a las regiones usando la variable de personeros presentes durante el escrutinio. Este mismo patrón se repite en varios departamentos, cuando se segregan los resultados con la variable de personeros en cada departamento. El impacto de esta distorsión seria suficientemente grande a nivel nacional, como para reducir los resultados de los votos a favor de Fuerza Popular en más de 147,897 votos.
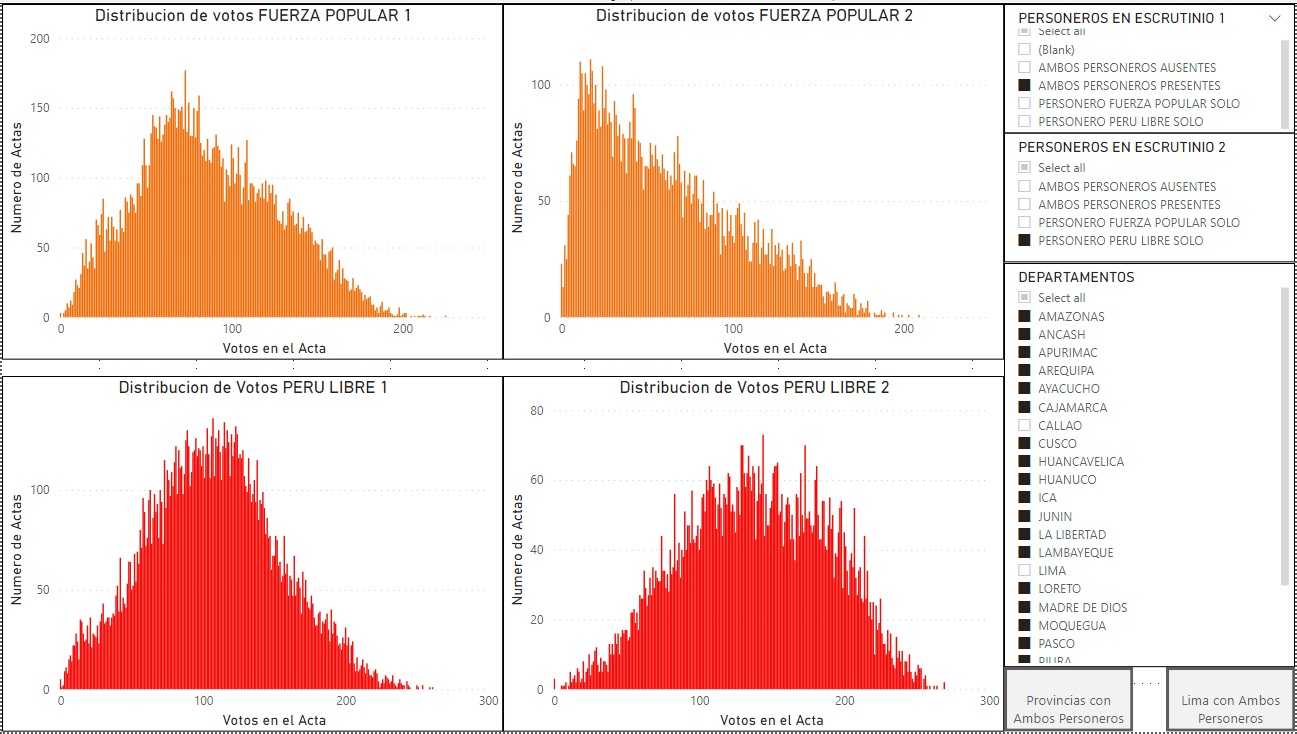
Figura Resumen: En Regiones sin Lima, la distribución de votos de Fuerza Popular no es consistente. Como lo muestra la siguiente figura, la distribución muestra forma normal cuando ambos personeros están presente (1), pero cuando únicamente el personero de Perú Libre está presente (2), la curva es extremadamente sesgada. Lo que no es consistente para la misma población regional, que muestra resultados diferentes cuando se segrega usando la variable de personeros presentes durante el escrutinio. El mismo patrón se muestra en detalle en varios departamentos.
Introducción
En las elecciones presidenciales Peruanas de la segunda vuelta 2021, se puede visualizar que la distribución estadística de los votos de Fuerza Popular, muestra distorsiones con una distribución muy sesgada con un número alto de mesas con votación muy baja para Fuerza Popular.
La ubicación geográfica (departamento, provincia, distrito, local) sería la única variable que explicaría estas distorsiones como producto de un fenómeno regional, donde la votación de Fuerza Popular resulta en un alto número de mesas con muy bajas votaciones en favor de Fuerza Popular.
El análisis presentado en este documento incluye una nueva variable: la información de los personeros que participaron en el escrutinio.
Las actas de cada mesa contienen secciones donde firman los miembros de mesa y los personeros presentes durante la instalación, escrutinio y sufragio de cada mesa.
Para este análisis se procesó y contabilizó la data de personeros durante el escrutinio de más de 85,816 mesas, que son más del 99% del total de las mesas que se habilitaron en las elecciones. Se pueden determinar cuatro casos diferentes para cada mesa:
- Ambos personeros de Fuerza Popular y Perú Libre estuvieron presentes durante el escrutinio.
- Únicamente estuvo presente el personero de Fuerza Popular durante el escrutinio.
- Únicamente estuvo presente el personero de Perú Libre durante el escrutinio.
- Ningún personero estuvo presente durante el escrutinio.
Total de personeros
Esta información revela patrones en los datos que no pudieron ser identificados previamente con la data publicada por la ONPE.
En la figura 1, se puede ver que Perú Libre pudo cubrir más de 36,149 mesas con sus personeros, mientras Fuerza popular solo pudo cubrir alrededor de 29,235 mesas con sus personeros durante el escrutinio de los votos.
También se observa que el número de mesas donde únicamente estuvo el personero de Perú Libre y no estuvo el de Fuerza Popular, fueron alrededor de 11,210 mesas. El número de mesas donde solo estuvo el personero de Fuerza Popular y no estuvo presente el personero de Perú Libre, fueron 4,296 mesas, en otras palabras Perú Libre tuvo más del doble de mesas cubiertas únicamente por un personero de un partido.
Hubo cerca de 24,939 mesas a nivel nacional, donde ambos personeros, de Perú Libre y Fuerza Popular, estuvieron presentes durante el escrutinio. Y finalmente, hubo 45,398 mesas donde no estuvieron presentes ambos personeros.
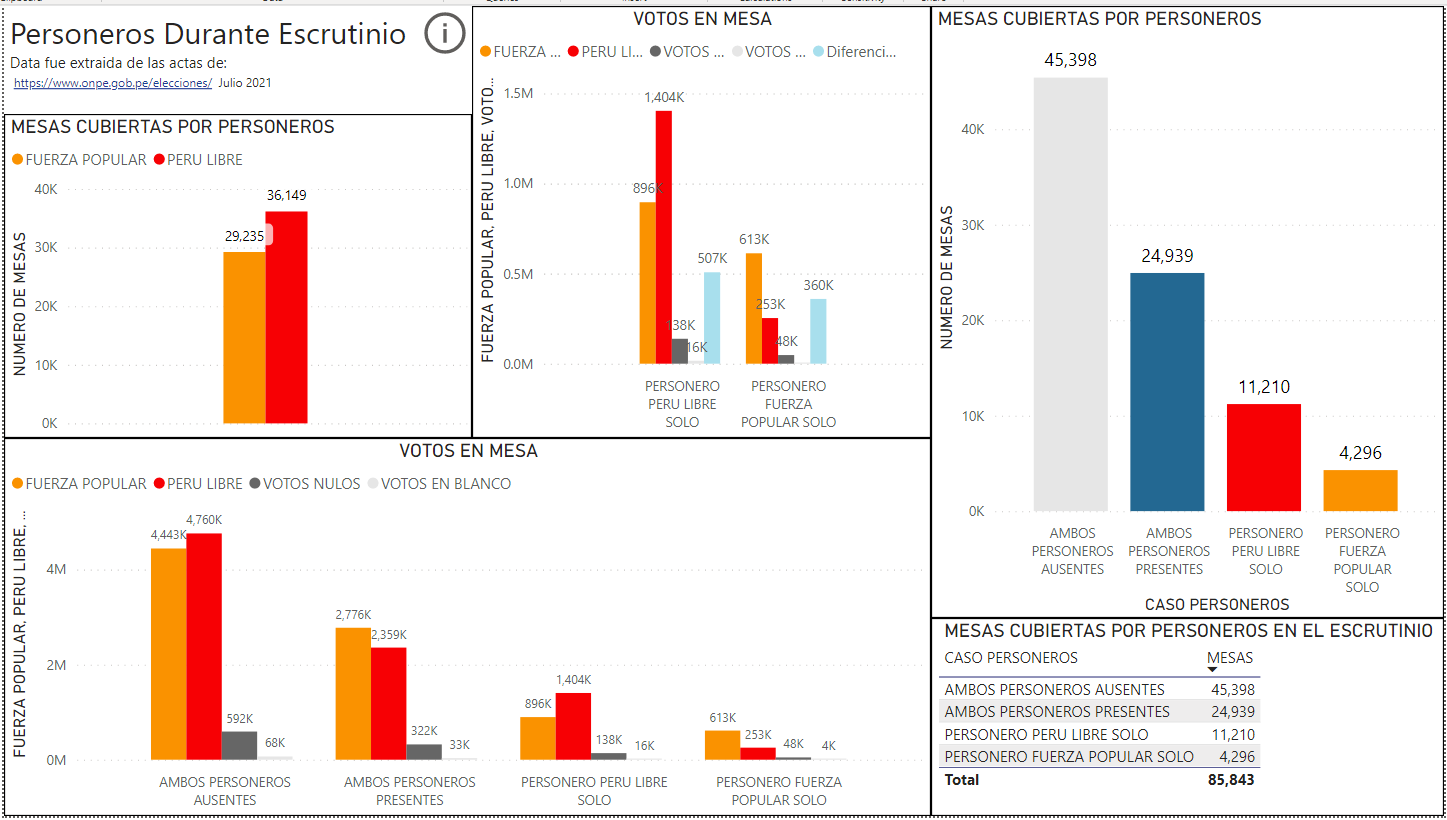
Figura 1: Personeros Durante el escrutinio
Distribución Normal
La curva de campana, mostrada en la figura 2, es el tipo de distribución para una variable que se considerada normal o Gaussiana.
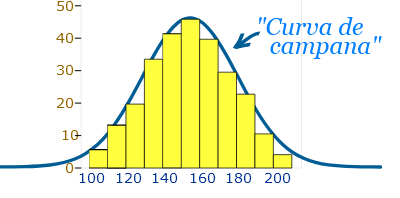
Figura 2: Curva Normal o de Gauss
Distribución de Votos a Nivel Nacional
A nivel nacional, la distribución total de los votos de Fuerza Popular no presenta una forma de curva de campana o curva normal, como se ve en la figura 3, mientras que la curva de distribución de votos de Perú Libre a nivel nacional, si presenta una forma de campana o forma de curva normal.
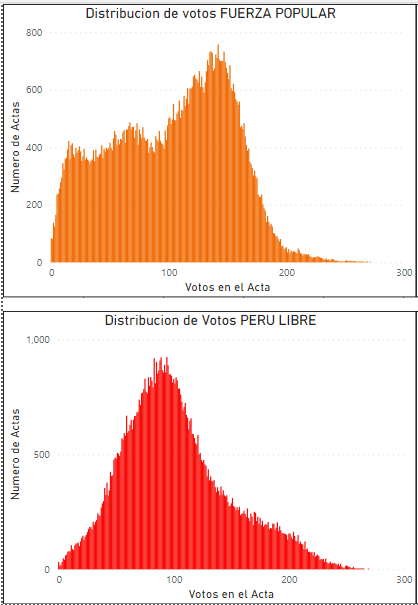
Figura 3: Distribución a nivel nacional de los votos en las mesas para las elecciones de segunda vuelta en Perú 2021. La curva de Fuerza Popular no es normal, mientras que la curva de Perú Libre si muestra una curva normal o gaussiana.
Lima versus Regiones sin Lima: Curva Normal versus curva Sesgada
En la Figura 4, se muestra la distribución de los votos de Fuerza Popular en Lima y en regiones sin Lima. Se puede identificar que en Lima y Callao la curva es normal, mientras que en Perú-sin Lima, la distribución de votos esta sesgada a la izquierda donde hay una cantidad grande de actas con votos muy bajos a favor de Fuerza Popular.
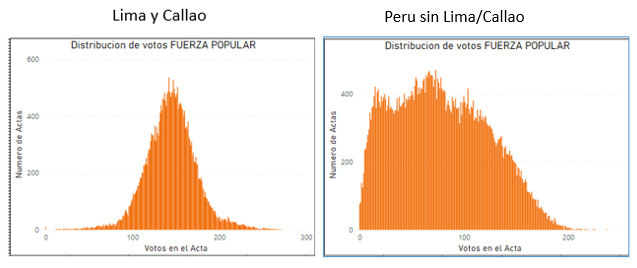
Figura 4: Comparación de la distribución de votos de Fuerza Popular en Lima/Callao versus las otras regiones del Perú sin Lima. La curva de Lima/Callao es definitivamente normal y la curva del Perú sin Lima presenta distorsiones.
Una explicación de este resultado propone que la población en ciertas regiones votó en forma diferente a Lima y esto resulto en una cantidad alta de mesas con votos muy bajos para Fuerza Popular.
Distribución en la región Lima es Normal
En la figura 5, se puede apreciar que en Lima la curva es normal para toda la región Lima/Callao y que seleccionando los casos donde los dos personeros estuvieron presentes, la curva también tiene una forma normal, lo que es consistente con la región geográfica de Lima y Callao.
Cuando ambos personeros están presente durante el escrutinio, el resultado de la votación es más fiable y exacta, ya que hay balances y chequeos de ambos personeros, razón por la que usamos este caso de personeros para poder comparar con los resultados totales en cada región.
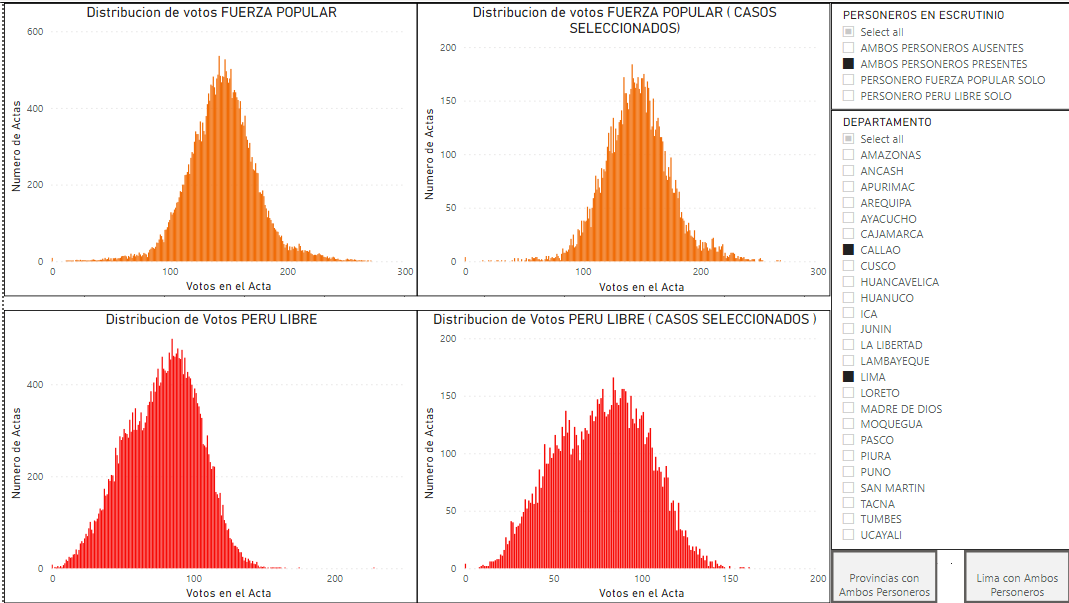
Figura 5: LIMA/CALLAO: Comparación de la distribución de votos en Lima versus la distribución de votos en Lima con solo mesas donde ambos personeros están presentes durante el escrutinio. Las curvas son consistentes en la región, sin importar que los personeros de ambos partidos estuvieran presentes durante el escrutinio.
Conflicto en la Distribución en regiones fuera de Lima: curva es sesgada y normal en la misma región
El patrón de curva sesgada es el que se esperaría en las regiones de Perú-sin Lima. Si se selecciona solo las mesas en departamentos del Perú-sin Lima, donde ambos personeros están presentes, se esperaría obtener la misma curva este sesgada a la izquierda, para que sea consistente con el comportamiento de la población de la misma región geográfica.
En la figura 6, se comparan las distribuciones de votos de las mesas en regiones del Perú-sin Lima. En el lado izquierdo se muestra la curva de todas las mesas de votos de Fuerza Popular en Perú-sin Lima, en el cual se nota que esta curva esta sesgada hacia la izquierda. En el lado derecho de la figura, se ha seleccionado solo las mesas donde los dos personeros de los dos partidos estuvieron presente en las mesas de Perú-sin Lima. En este caso, la curva ya no está sesgada y se convierte en una curva normal, que es opuesto a resultado de las regiones que tienen una curva sesgada a la izquierda.
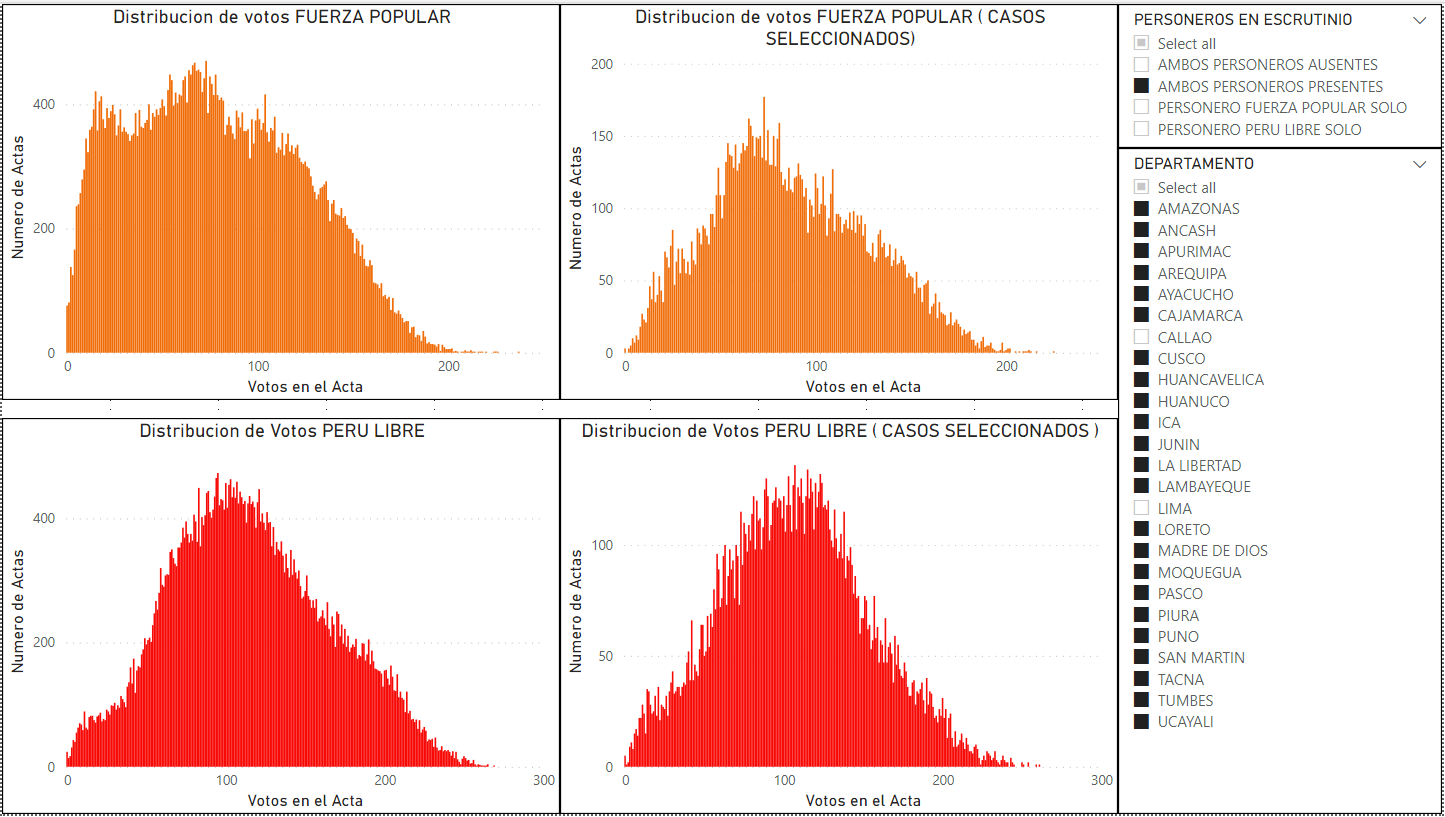
Figura 6: DEPARTAMENTOS EXCLUYE LIMA/CALLAO. Comparación de la distribución de votos en departamentos fuera de la capital versus la distribución de votos de la misma región con las mesas donde ambos personeros estuvieron presentes durante el escrutinio. Las curvas de votos de Fuerza Popular esta sesgada a la izquierda y cuando se seleccionan solo las mesas con ambos personeros presentes durante el escrutinio, la curva es peculiarmente normal. El comportamiento no es consistente en la región.
Las dos curvas de Fuerza Popular de la figura 6 no son consistentes con la ubicación geográfica de Perú-sin Lima, porque son diferentes dependiendo de la variable de personeros presentes durante el escrutinio.
En la figura 7, cuando se selecciona y compara con las mesas donde solo hubo personeros de Perú Libre se ve evidentemente un resultado muy diferente, para Perú-sin Lima. La curva extremadamente segada a la izquierda, que evidencia baja votación para Fuerza Popular.
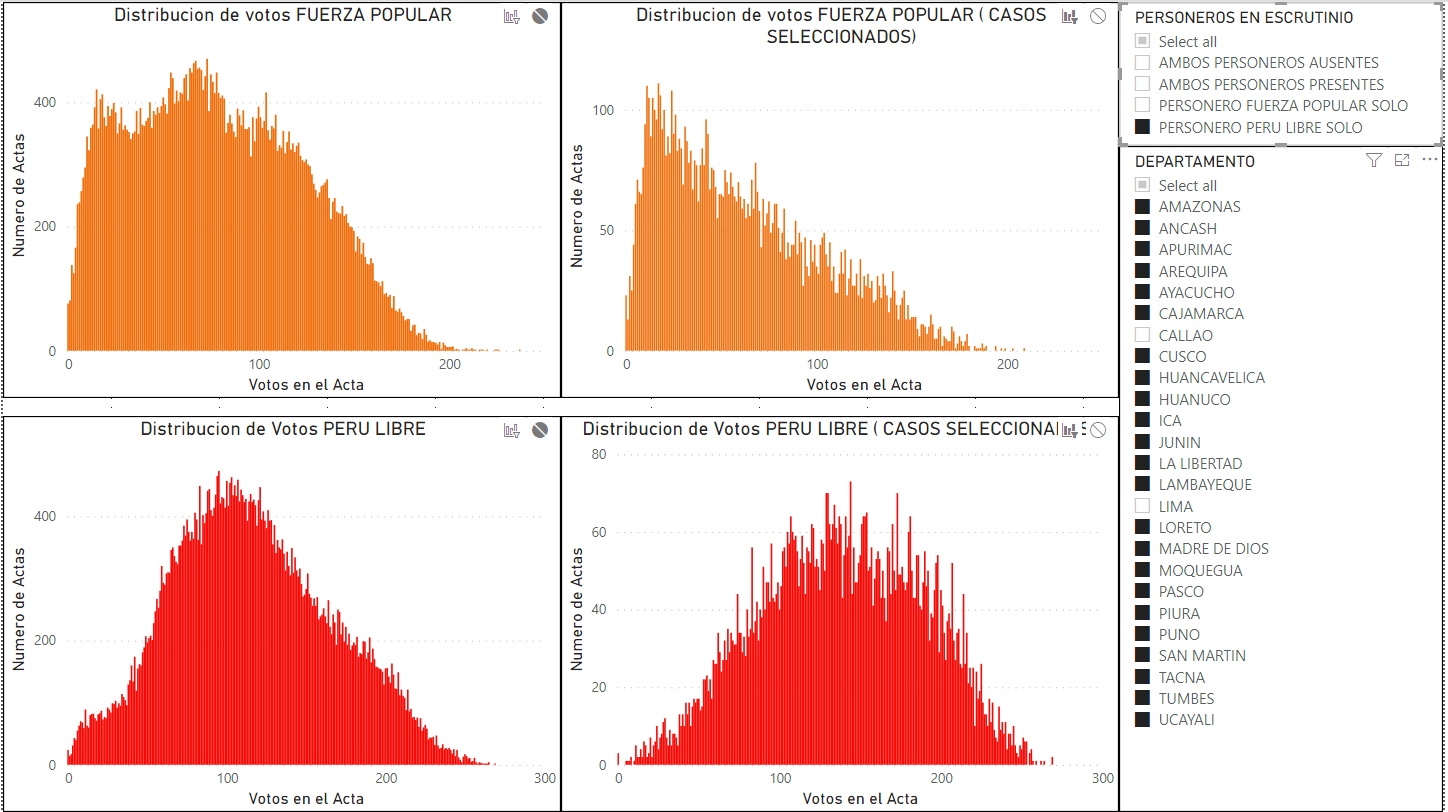
Figura 7: Comparación de la distribución de votos en regiones sin Lima versus la distribución de votos en las mismas regiones sin Lima con solo las mesas donde solo estuvo el personero de Perú Libre presente durante el escrutinio. Los votos de Fuerza Popular están sesgados a la izquierda cuando solo está presente el personero de Perú Libre.
Distribución en los Departamentos
Teniendo un resultado no consistente en regiones fuera de Lima, donde la curva total es sesgada, pero es normal cuando los dos personeros están presentes, el siguiente paso es comparar las mismas curvas a nivel de departamento.
En la figura 8, se puede observar las curvas a nivel de cada departamento y en especial en los departamentos que se señalan con un recuadro rojo: Cusco, Cajamarca, Puno y otros muestra un sesgo pronunciado a la izquierda.
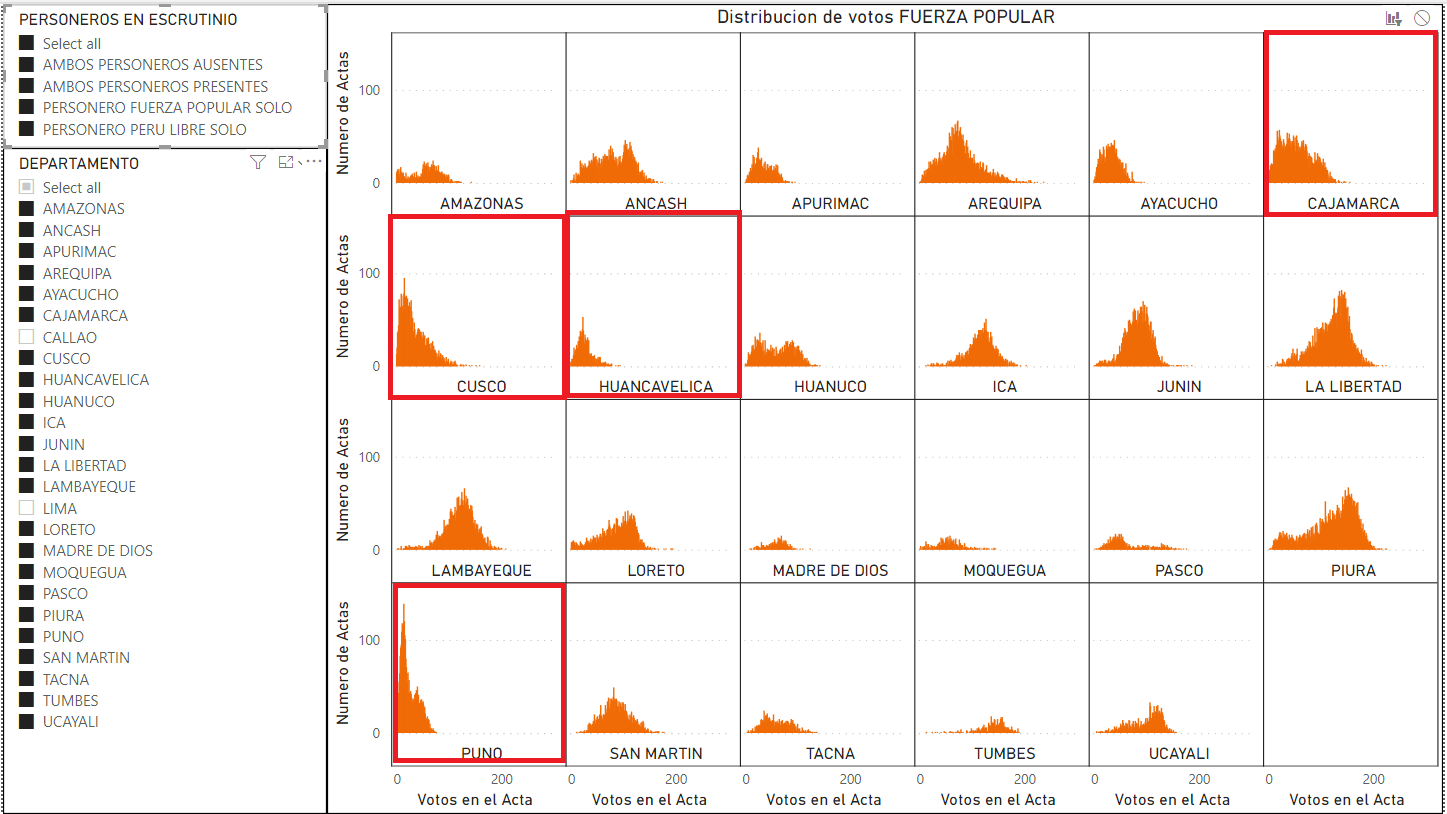
Figura 8: Distribución de votos de Fuerza Popular de cada departamento. Muestra varios departamentos con curvas sesgadas a la izquierda.
En la figura 9, cuando se selecciona solo las mesas donde están presentes ambos personeros, las curvas tienden a ser normales, tal como se ve en el agregado de las regiones. Note la diferencia de los departamentos de Cajamarca, Cusco y Puno con formal normal con la de la figura 8, donde los mismos departamentos muestran una curva de votos sesgadas.
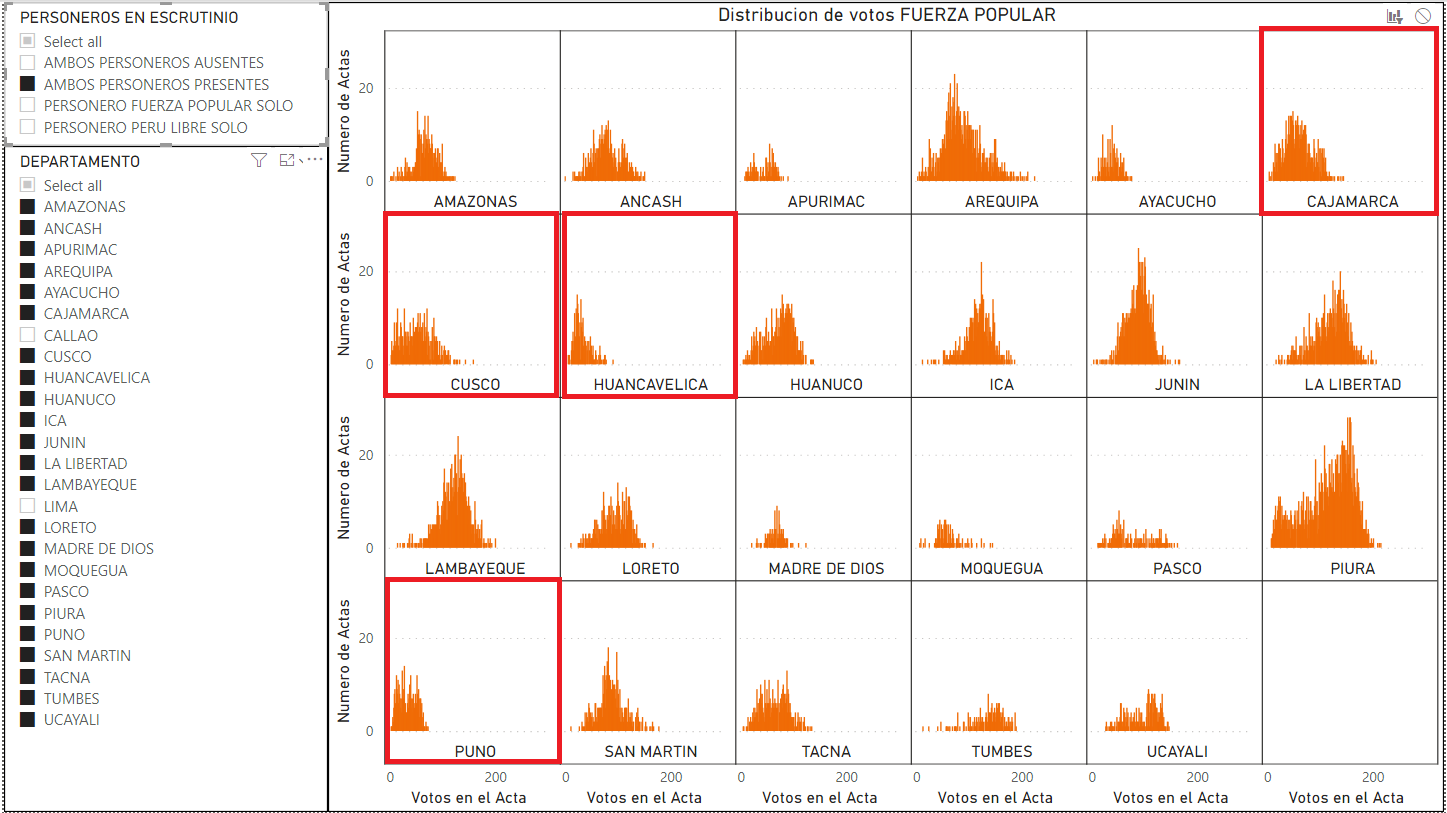
Figura 9: Distribución de votos de Fuerza Popular de cada departamento con mesas donde estuvieron presentes ambos personeros de Fuerza Popular y Perú Libre durante el escrutinio. Las curvas no están sesgadas a la izquierda, las curvas muestran un patrón de curva normal.
Y por último, en la figura 10, cuando se seleccionan solo las mesas donde Perú Libre tuvo un personero y Fuerza Popular no estuvo presente, las curvas se distorsionan notablemente hacia la izquierda que muestra una cantidad elevada de votos bajos para fuerza popular.
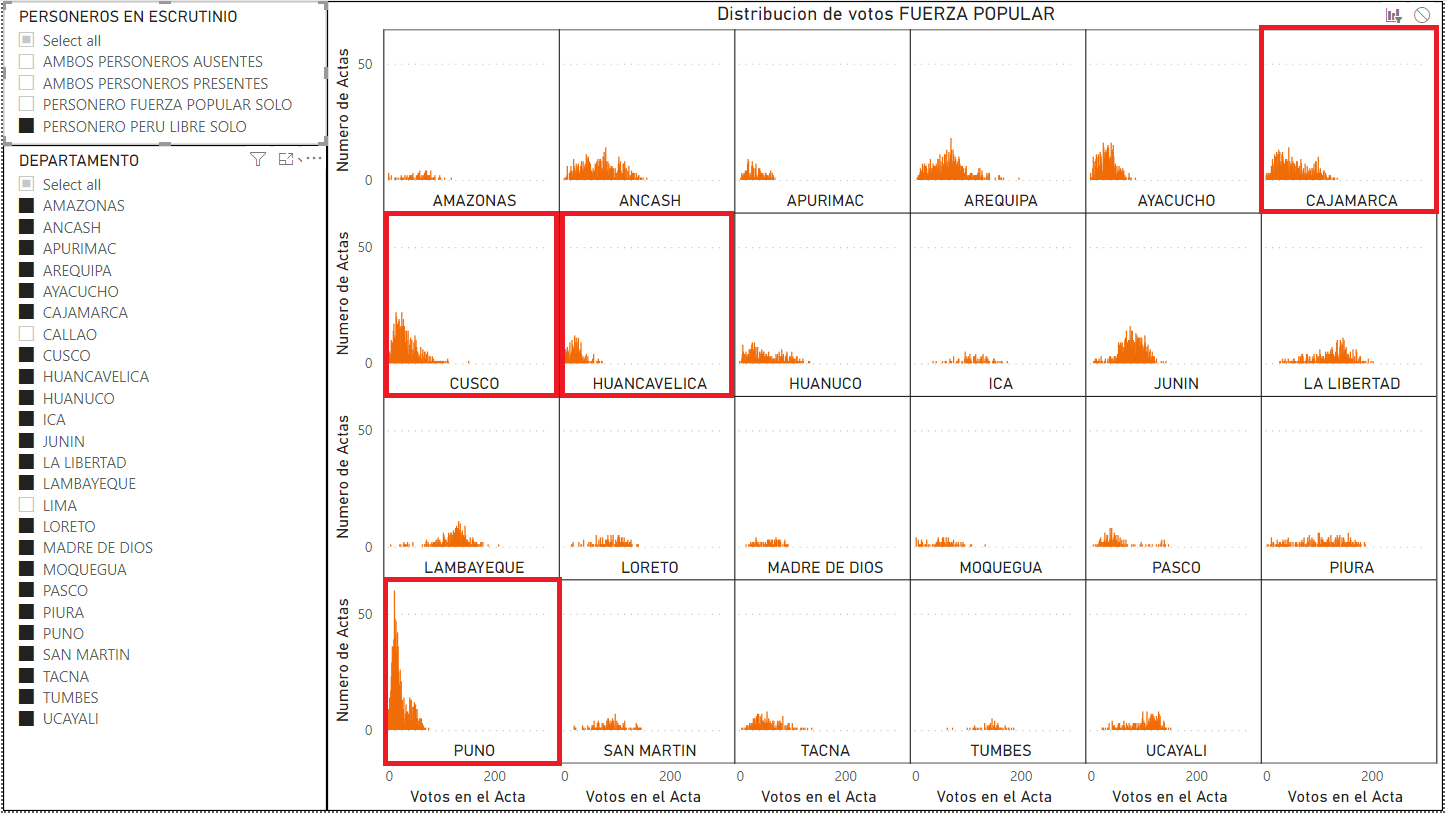
Figura 10: Distribución de votos de Fuerza Popular de cada departamento con mesas donde estuvieron presentes solo personeros de Perú Libre durante el escrutinio. Las curvas muestran un sesgo muy amplio hacia la izquierda.
Caso cuando no hay personeros en la mesa
El caso cuando no hay personeros de ningún partido en la mesa durante el escrutinio debería ser examinado con cuidado. En el entrenamiento a personeros de Perú Libre se recomendó a sus seguidores a ser miembros de las mesas donde les tocaba sufragar, tal como lo dice en el documento de entrenamiento de Perú Libre en la Figura 11.
Si este fuera el caso, el impacto de las distorsiones incluiría también los casos donde no hubo personeros durante el escrutinio de las mesas, pero podría haber influencia escondida de seguidores de Perú Libre como miembros de mesa, sin personeros en mesa.
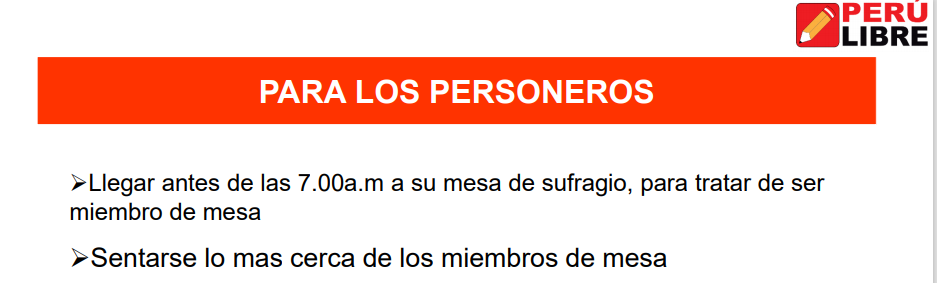
Figura 11: Documento Oficial de capacitación de personeros de Perú Libre.
Publicado en Abril de 2021 en la página web oficial de Perú Libre:
http://perulibre.pe/wp-content/uploads/2021/04/capacitacion-personeros.pdf
Impacto en los resultados finales
El impacto de los personeros fue muy determinante en las elecciones de segunda vuelta en número total de votos. En la figura 12, se puede ver el resultado de la simulación de un escenario donde se cuentan las mesas que tuvieron dos personeros de ambos partidos: Perú Libre y Fuerza Popular o ningún personero en mesa, eliminando las mesas donde solo un personero de un partido está presente. Fuerza Popular obtiene una ventaja de 103,657 votos. Lo que resulta en una diferencia de más de 147,897 votos si se agrega la diferencia de 44,240 votos a favor de Perú Libre. Esto demuestra que el factor personeros es importante y determinante para el resultado final de las elecciones a nivel nacional.
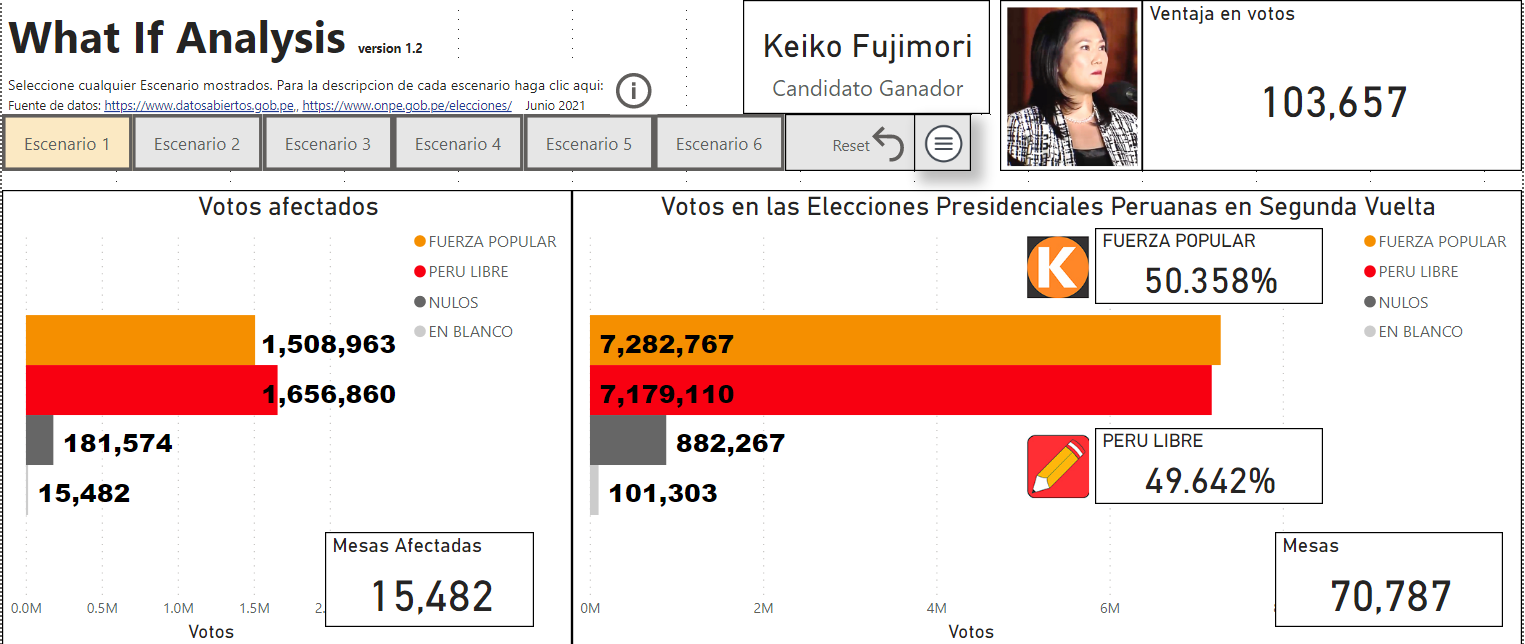
Figura 12: Escenario donde se eliminan las mesas donde personeros de Perú Libre estuvieron únicamente en la mesa y se eliminan las mesas donde los personeros de Fuerza Popular estuvieron únicamente en la mesa.
Conclusion
Con esta nueva información se abren preguntas acerca de las regiones sin incluir Lima/Callao:
¿Por qué los votos a favor de Fuerza Popular no son consistentes dentro de cada región?
¿Por qué las curvas son diferentes cuando se comparan los votos en los casos que hay dos personeros presentes versus cuando únicamente está el personero de Perú Libre presente dentro de cada región?
La curva de votos a favor de Fuerza Popular es sesgada en algunas regiones. Pero la curva no es sesgada cuando se toma en cuenta mesas donde ambos personeros están presentes durante el escrutinio en la misma región.
Cual otra variable, fuera de la de personeros, podría explicar este patrón irregular de resultados de las votaciones cuando esta el personero de Perú Libre?
Estos datos encontrados, rechazarían la hipótesis que la distribución de los votos de Fuerza Popular en regiones al interior del país esta sesgada debida solo a que la población en estas regiones tiene un patrón de votación diferente. Este análisis de los datos de los personeros, evidencia resultados en la votación diferente o inconsistente para la misma población regional, cuando ambos personeros están presentes y cuando solo el personero de Perú Libre está presente. Los resultados de la votación no son consistentes para el mismo departamento o en la misma región. Dependiendo del caso de personeros presentes, la curva es sesgada y en el otro caso la curva es normal.
La variable de personeros durante escrutinio explica este sesgado mejor que la variable geográfica y es finalmente la que determina el resultado final de los votos de Fuerza Popular. Estas distorsiones o sesgado a nivel regional se agregan y resultan en distorsiones a nivel nacional.
Como se muestra en el análisis de escenarios, estas distorsiones tienen un impacto determinante en el resultado final se las elecciones. Lo que resultaría, en caso de eliminar casos de mesas con personeros únicos de ambos partidos, en una ventaja a favor de fuerza popular de 103,657 votos.
Por esto hace necesario hacer una investigación más amplia, tomando en cuenta los casos expuestos para determinar la validez de los resultados.